Predicting a pandemic: How AI helped predict COVID-19’s twists and turns
When it was confirmed that animals, from white-tailed deer to red foxes, could contract and spread COVID-19, it dealt a huge blow to hopes that the disease could eventually be eradicated. For Dr Barbara Han, it came as no surprise.
- 28 March 2022
- 8 min read
- by Linda Geddes
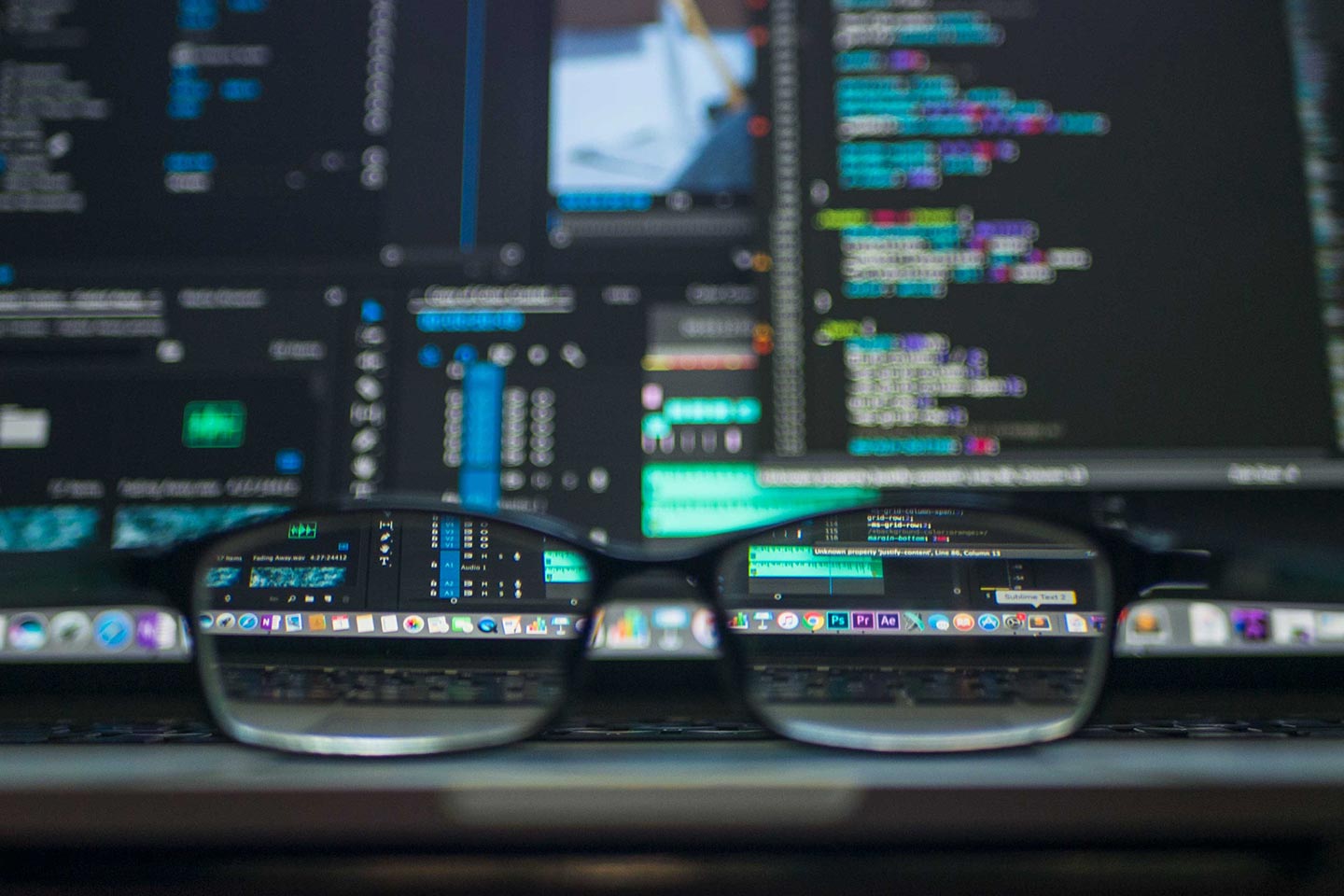
Barbara Han’s introduction to biology saw her immersed in Costa Rican rainforests dripping with life. As she collected amphibians from the tree trunks, pools and leafy undergrowth, and tried to make sense of how their biological characteristics affected their susceptibility to novel threats, she became increasingly aware of the challenge of collecting enough data points to make useful predictions.
Han and her colleagues are developing artificial intelligence that learns from ecological, biogeographical and public health data to identify which species are likely to harbour viruses that could spill over to humans.
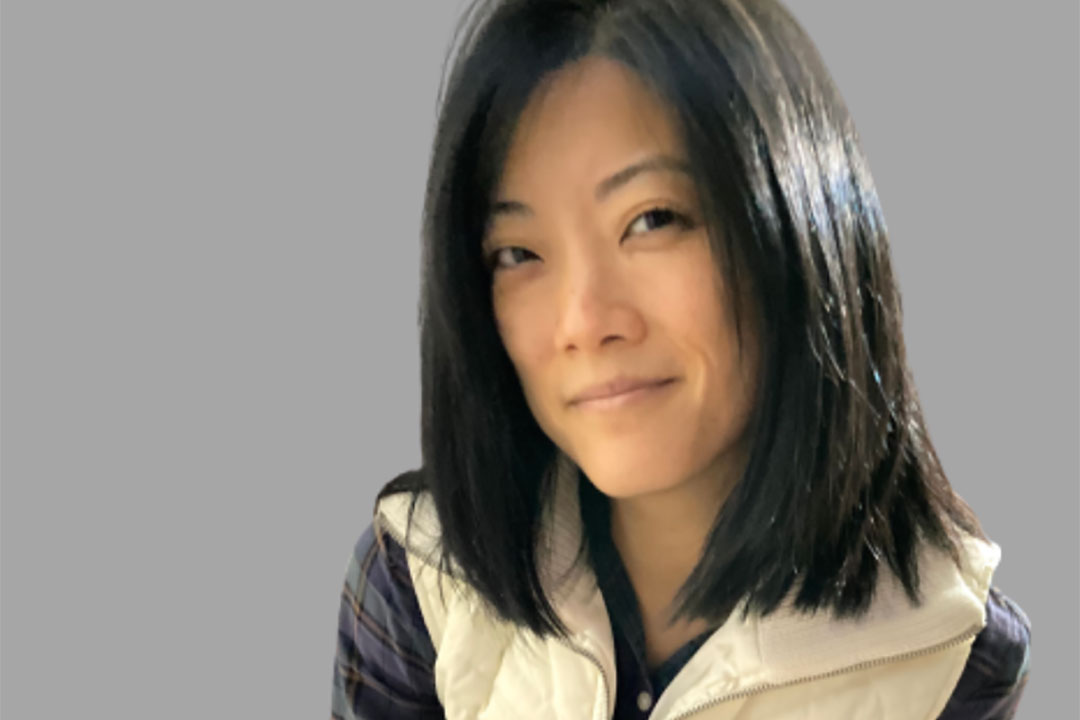
Several decades on, her day-to-day laboratory work couldn’t be further away from those life-drenched rainforests: “It is very computer based,” said Han. “We spend a lot of time tidying up datasets, removing typos, and making sure there's nothing like an extra zero added to a value that would make it totally nonsensical.” Yet her goal of using biological data to make actionable predictions about the threat of diseases remains the same.
Similar to how internet search engines and online retailers use algorithms to predict what individuals would like to read or purchase by learning from what they’ve searched for before, Han and her colleagues are developing artificial intelligence that learns from ecological, biogeographical and public health data to identify which species are likely to harbour viruses that could spill over to humans, and where such spillover events might occur. Such predictions could help to target disease surveillance efforts more strategically and pre-empt outbreaks, meaning they’re less likely to trigger another pandemic.
Biology is awash with data – from genetic blueprints of different species, to structural information about their proteins, to ecological data about their habitats, which foods they consume, or how many offspring they have.
In addition to collaborating on the collection of precious new data, Han’s team at the Cary Institute of Ecosystem Studies in New York State, US, combines existing datasets and deploys machine learning algorithms to comb through them and make broader predictions about species’ susceptibility to disease and potential to transmit it.
One of their early successes was the development of a model to predict which bat species are most likely to transmit Ebola and other filoviruses. Understanding this could help to prevent future outbreaks in humans.
Bats are a prime suspect for harbouring Ebola and triggering human outbreaks, but although various species have tested positive for filovirus antibodies, Ebola has never been isolated from a live African bat.
The predictions implicated animals like deer and red foxes that are in our backyards. How can we hope to get ahead of this and stamp it out?
To train their model, Han’s team created a database of physiological and ecological traits associated with those bat species already known to carry filoviruses. The model was then able to trawl through data from 1,116 other bat species to predict other potential hosts. Those it identified were far more geographically widespread than expected – found not only in sub-Saharan Africa, but across southeast Asia, central and South America.
Soon after publishing this work, Han was contacted by a separate research group that had been collecting biological samples from a species that ranked number five on their list – a nectivorous bat found in the Yunnan province of China. “It is not in the range that we expect spillovers, but it was a hot spot on our predictive map,” Han said. “They had found a bat carrying a novel filovirus in its lungs – which was also quite disturbing, because it suggested transmission may be a bit different to other filoviruses which are thought to spread through contact with infected body fluids. It was passive validation that our model was correct.”
When the COVID-19 pandemic hit, Han’s team pivoted their research towards trying to understand this novel coronavirus. But there was a major obstacle in their path: “You can't make predictions if you don’t have data, and at the outset of COVID-19 that’s exactly what we didn’t have,” said Han.
Even so, the team felt that they had a useful skills set to contribute, so they went back to first principles and asked themselves what was known about this new virus.
“None of these things are surprising, but it is still terrible to be right. Watching the media on the one screen and seeing that now snow leopards – which were also very high on our list – not only get infected but die from it. Knowing that we were right about something like that is awful.”
“We knew that the pathogen was transmitting extremely rapidly, and it was animal-borne, so the chances of it spilling back into an animal were quite high,” said Han. “We thought, maybe the best thing we can do at this point is to ask what are the kinds of animals that are at the highest risk of not just acquiring COVID-19 from humans and dying from it, but becoming wild reservoirs of the virus?”
Initially, they focused on identifying species that possessed ACE2 receptors – the key the virus uses to enter and infect cells. “We quickly figured out that anything with a backbone is likely to have some version of ACE2, which is somewhat the opposite of data sparsity and not helpful,” Han said.
So they turned to structural modelling: using amino acid sequence data for ACE2 from various species to predict how these molecular building blocks would fold into a 3D shape, and using this to estimate how tightly SARS-CoV-2 would bind to it.
To expand these predictions to thousands of additional species for which sequence data was unavailable, they trained a machine-learning model to use other biological data from these species to predict how well SARS-CoV-2 was likely to bind to their ACE2 receptors, and therefore how likely they were to be infected.
Have you read?
“ACE2 is very important for regulating homeostasis by controlling things like blood pressure, which is central to how an animal functions, which means its connection to other biological traits are likely to be more tightly coupled than other, less important characteristics,” Han explained.
Their model identified hundreds of mammals that were likely to be highly susceptible to SARS-CoV-2 – many of which live in close proximity to humans. Some of them, such as cats, mink, and bats, came as no surprise, because real world infections had already been documented. Others, such as water buffalo and deer, were less expected.
“Investing in upstream prevention actions to remove the tinder, as opposed to putting out a wildfire once it has started, is the most economically sound decision that we could make. But it is a hard sell – it is really difficult to convince somebody that you have a wildfire problem, before seeing any evidence of a fire.”
To say that Han was unhappy with some of these predictions is an understatement: “It was a terrible finding,” she said. “It wasn’t that it was bad data, but the predictions implicated animals like deer and red foxes that are in our backyards. How can we hope to get ahead of this and stamp it out? It seemed highly likely that the pathogen would be able to establish itself in a common and highly abundant species – possibly one that is farmed.”
Some of their predictions have already come to pass, such as the discovery that white-tailed deer can become infected and also spread the virus. Han said: “White-tailed deer are one of the most common species in the western hemisphere, so we weren't surprised at all to find that it had become infected by humans. And the confirmatory findings on the deer mouse, we weren't surprised. The red fox, we weren’t surprised.
“None of these things are surprising, but it is still terrible to be right. Watching the media on the one screen and seeing that now snow leopards – which were also very high on our list – not only get infected but die from it. Knowing that we were right about something like that is awful.”
However, the model hasn’t been right about everything. For instance, it gave a high probability that pigs could be infected, but experiments suggest this doesn’t happen. Similarly, it assigned cattle a moderately high probability of infection, but although experiments have suggested they are susceptible to the virus, so far there is no evidence that they can transmit it to other cattle.
Understanding these mispredictions can be as illuminating as the predictions the model gets right. “It could maybe suggest you haven't included a piece of data that's really important,” said Han. Yet, unpacking precisely how the AI reached its conclusions isn’t straightforward. “It's not like the machine paints a picture for you,” Han explained. “It gives you an output, and it is then up to biologists to look at that and interpret what it means.”
Identifying potential animal hosts of new diseases has practical implications, such as identifying where surveillance efforts should be targeted, or whether certain species, and the humans that are in regular contact with them, should be prioritised for vaccination.
However, Han’s ultimate goal is to develop a broader model that could help predict how any zoonotic disease would interact with any animal species in any given environment. Doing so could enable policymakers to identify the fastest and most cost-effective ways of reducing the risk of animal diseases jumping into human populations, and vice versa, where to target surveillance efforts to rapidly identify outbreaks, and how best to prevent such outbreaks from spiralling out of control.
Doing so, won’t be easy, but almost as challenging is persuading funders that such systems are necessary. Han said: “Investing in upstream prevention actions to remove the tinder, as opposed to putting out a wildfire once it has started, is the most economically sound decision that we could make. But it is a hard sell – it is really difficult to convince somebody that you have a wildfire problem, before seeing any evidence of a fire.”