How AI is transforming health care in Kenya amid rapid innovation
In the health care sector, expectations of what artificial intelligence can achieve are running high - and in Kenya, innovators are already making waves.
- 30 October 2024
- 6 min read
- by Claudia Lacave
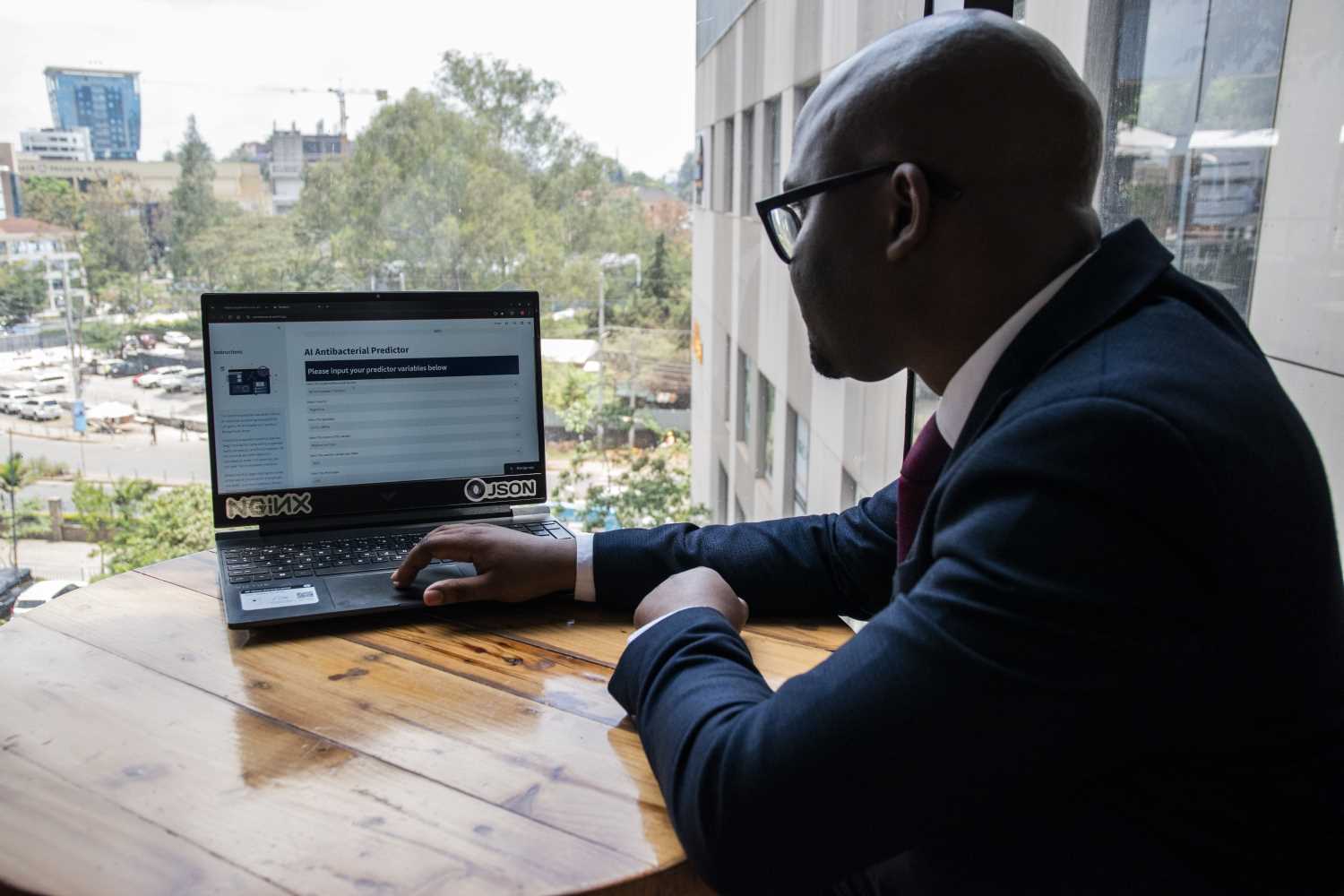
Since the launch of ChatGPT in 2022, artificial intelligence (AI) has become a hot topic, generating significant excitement across various fields. In the health care sector, expectations are especially high. In Kenya, while the government is working on regulations, innovations are already forging ahead.
Click on the ‘AI Antibacterial Predictor’ tab, then select bacterium, country, sample type, year and antibiotic. After that, click on “AI Model Result.” In just a second, the Antimicro.ai platform estimates the likelihood that the organism will be resistant to the selected drug.
AI technology can often seem unfathomable, yet solutions to real problems lie within these lines of code. Dr Fredrick Mutisya, who co-founded the platform alongside Dr Rachael Kanguha, knows this well: “We use AI to help doctors make informed antibiotic prescriptions, thereby combatting antimicrobial resistance (AMR).”
The Kenyan government has embarked on developing its national AI strategy, and Nairobi recently hosted African Ministers of Information in April this year, where they reaffirmed the crucial role of digitalisation and digital infrastructure in modern economies. Two public consultations, organised by the tech innovation firm Qhala, convened key health sector stakeholders to discuss the needs and opportunities AI presents. Professionals, regulators, innovators and researchers reached a consensus on the necessity for a collaborative, people-centred approach rooted in Africa.
AI could also help address the continent’s health care worker shortage. Currently, Africans have, on average, three doctors per 10,000 people, compared to 34 in high-income countries. Rather than replacing professionals, AI could, for instance, enhance the expertise and capacity of existing health staff, enabling them to deliver more precise and effective care.
“The advantage of a large general language model is that once operational, it could help health professionals in remote or under-resourced areas […] make informed decisions on specific cases. But for this to become a reality, it requires a lot of data and is a very expensive process.”
- Dr Beatrice Gatumia, programme director at AMREF
Informing medical and policy decisions
Dr Mutisya attended both meetings to present his agenda. A 35-year-old physician at Narok Hospital in western Kenya, he used the downtime during the COVID-19 pandemic to teach himself solid programming skills. He has since continued his studies, earning a master’s degree in epidemiology from Moi University in Kenya and an online master’s degree in data science from the International University of Applied Sciences in Germany.
When he learned about the first AMR Data Challenge organised by the NGO Vivli and the Wellcome Foundation, he didn’t hesitate – he immediately thought of AI. Using Pfizer’s antibacterial surveillance data, collected from 2004 to 2021, he and Dr Kanguha developed a platform to predict antibiotic resistance. Antimicro.ai thus enables an initial prescription for patients while awaiting more precise laboratory results.
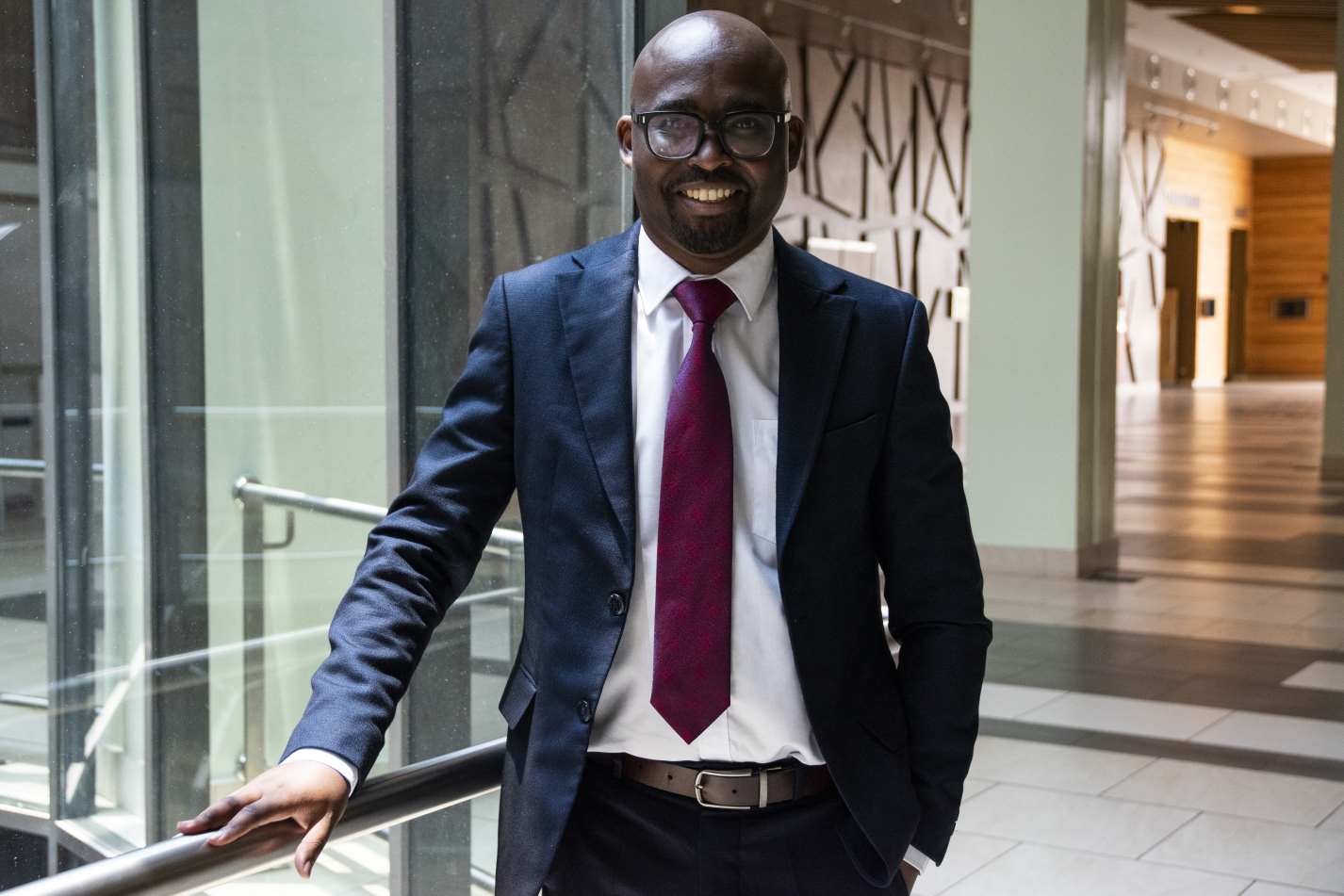
Credit: Claudia Lacave/Hans Lucas
The US pharma company’s database, which contains over 850,000 samples from 83 countries, covering 341 bacterial species and 38 different drugs, is a powerful computational asset for AI. Previous studies using this data for antimicrobial resistance (AMR) research were based on a median of 2,630 samples.
Antimicro.ai has estimated that certain bacteria exhibit resistance rates over 50% in some populations, with projections reaching up to 80% by 2030. Tools like this one could help identify new resistance trends, enabling governments to take proactive measures through evidence-based national guidelines. It could also support more effective planning for drug supplies, preventing stock-outs and ensuring better resource allocation.
Open-source
Yet Pfizer’s data has its limitations: “We’ve noticed that this data is useful for setting global policies, but when it comes to hospital-level predictions, practitioners often need more granular data. Pfizer’s data is depersonalised and stops at the country level, so some prefer to use their own equipment,” explains the doctor from Narok.
The winning team of the Vivli Grand Prix has therefore made the web application accessible to anyone who wants to use their own data, without it being stored or reused by the program. The open-source code has drawn interest from other professionals, like a PhD student from Kisii in western Kenya, who, with guidance from the team, used AI to identify patients in rural communities who needed resistance testing, achieving a 90% accuracy rate.
Beyond country-level anonymisation, the Pfizer data’s provenance also poses a challenge. The samples are sourced 48% from Europe and Central Asia, 21% from North America, 11% from South America and the Caribbean, 10% from East Asia and the Pacific, 5% from the Middle East and North Africa, and only 2% each from sub-Saharan Africa and South Asia. The reality is that Africa lacks sufficient health data to fully harness the potential of AI at this point in time.
Have you read?
Large language model needed for Africa
“The advantage of a large general language model is that, once functional, it could assist health care professionals in remote or underserved areas – such as northern Kenya – in making informed decisions on specific cases. However, making this a reality requires vast amounts of data and is an extremely costly process,” explains Dr Beatrice Gatumia.
Dr Gatumia, a programme director at AMREF, Africa’s leading public health NGO, believes that developing a large language model (LLM) tailored for Africa – an AI system capable of understanding and generating text – would be valuable but challenging. Such an undertaking would need a large volume of health data and the expertise of health professionals to label and train the model.
Currently, no such LLM exists on the continent, and most African countries lack open-access health databases. Using LLMs trained on data from other regions poses risks, as it could lead to diagnostic errors, given that population histories and biological characteristics influence responses to treatments.
Dr Gatumia points out that, despite the fact that ChatGPT does not have a specialist health care function, some practitioners are already using it to support medical decisions. Given the continent’s competing priorities, she raises a question: “Should we, for example, take an LLM trained on obstetric ultrasound data from Europe or America and adapt it, in a way, for Africa?”
This brings up the need for infrastructure to secure data storage, ownership, and protection, as well as to ensure equitable access to this technology – which demands hardware, electricity, and internet connectivity. Strong ethical guidelines for AI in health care are crucial, especially concerning accountability for medical errors.
The Kenyan government is aiming to finalise its national AI strategy by the end of the year. In April, the Bureau of Standards published a draft code of practice to regulate AI use in alignment with citizens’ rights. Meanwhile, new applications are emerging. Drs Mutisya and Kanguha have reapplied to Vivli’s challenge, this time incorporating insights from scientific research, including reasons for resistance, recommended dosages and potential side effects.