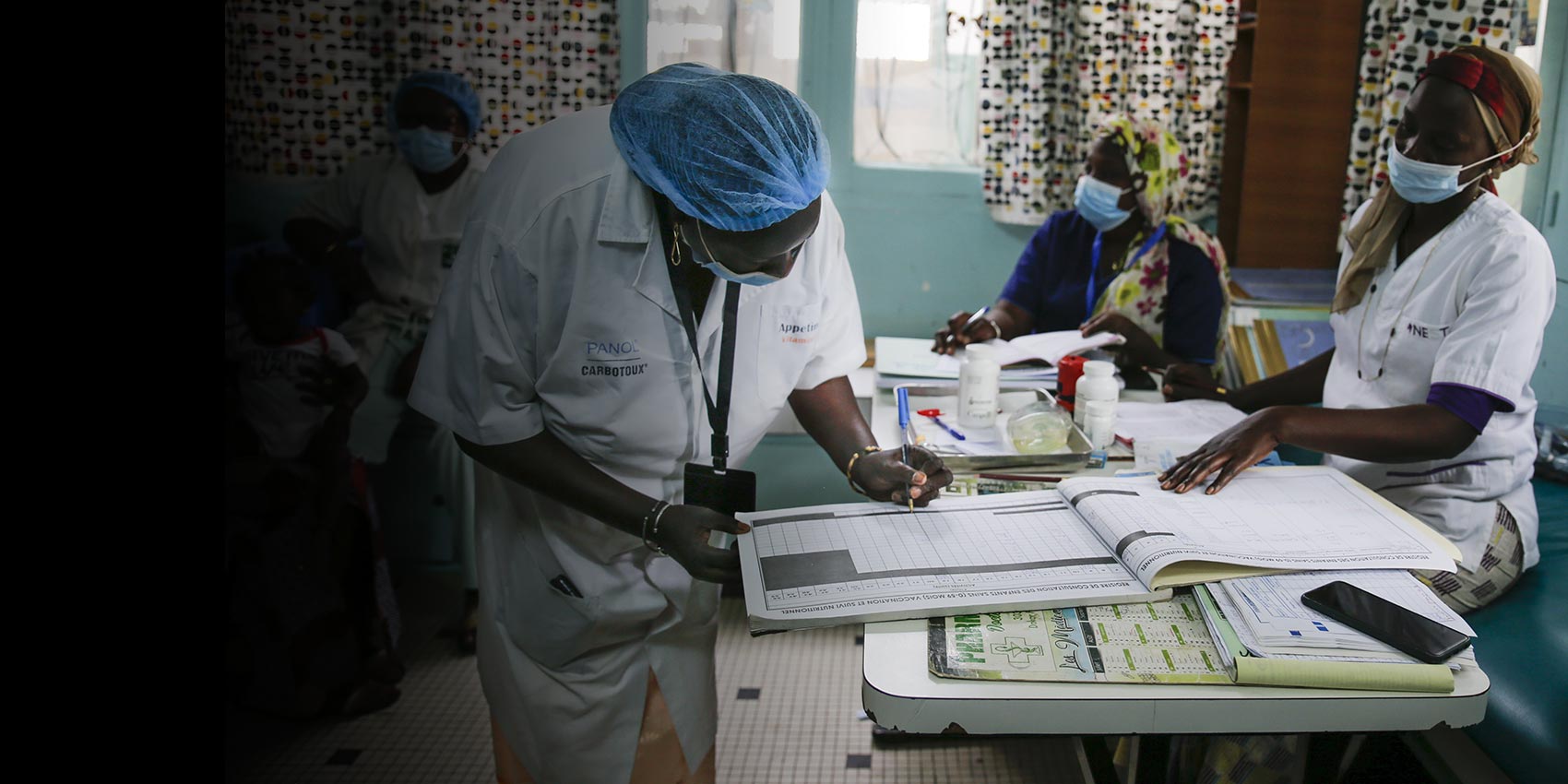
Back to
How “data poverty” could worsen health inequalities during the pandemic and beyond
Big data has the potential to change society for the better, but this opportunity is being undermined by a failure to collect data from across societies and make it publicly available.
14 October 2020
Back to
How “data poverty” could worsen health inequalities during the pandemic and beyond
Big data has the potential to change society for the better, but this opportunity is being undermined by a failure to collect data from across societies and make it publicly available.
14 October 2020